PhD defense of François-Xavier CARTON on 12/13/21

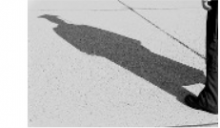
PhD defense of François-Xavier CARTON from TIMC GMCAO team on december, the 13th, at 3:30pm:
« Image segmentation and registration
using machine learning for brain shift compensation
in image-guided neurosurgery. »
Jury:
- Matthieu CHABANAS, Senior lecturer, UGA (Grenoble, France), Director
- Jack NOBLE, Assistant professor, Vanderbilt University (Nashville, USA), Co-director
- Antoine SIMON, Senior lecturer, Université de Rennes 1 (Rennes, France), Reporter
- Marc MODAT, Senior lecturer, King's College London (Londres, Royaume-Uni), Reporter
- Michel DESVIGNES, Professor, Grenoble INP (Grenoble, France), Examiner
- Jocelyne TROCCAZ, Research director, UGA (Grenoble, France), Examiner
- Thomas LANGO, Chief scientist/Research director, SINTEF (Trondheim, Norgève), Guest
Key Words:
Brain-shift, image-guided neurosurgery, image segmentation, image registration, deep learning.
Abstract:
In brain tumor resection surgery, a preoperative magnetic resonance (MR) image is used for surgical planning and guidance. Brain tissue displacement during surgery, known as brain shift, severely limits image guidance with the preoperative MR (pMR), which no longer reflects the location of tissue and anatomical structures accurately. This can be mitigated by registering the pMR with intraoperative data, ie. updating the pMR so that it matches images acquired during surgery. Ultrasound (US) imaging has several benefits compared to other intraoperative imaging modalities such as MR. In particular, it has fast acquisition times and do not require moving the patients, which makes the surgery longer. Thus, there is growing interest in using US imaging in brain surgery, despite the low image quality.
While traditional registration methods have been proposed, deep learning (DL) are being actively researched as they have proven successful and achieve state-of-the-art results in many domains such as medical image segmentation, and more recently, image registration. DL models are complex models with parameters learned with data, with many applications. However, there are few studies proposing DL models for segmentation of intraoperative US (iUS) images of the brain, or for registration of the pMR image with iUS images.
We start with the segmentation of iUS images of the brain. This is can be used for several purposes, such as guiding a registration model, which is the main objective of this thesis. We first focus on the segmentation of the resection cavity, which is essential to model the tissue displacement due to tissue removal. Next, we address tumor tissue segmentation, which is more challenging but can be used not only for registration but also to determine if tissue resection is complete. The results show that DL models can successfully segment the resection cavity and tumor tissue in US images. Then, we train a multi-class segmentation model, that segments several structures at the same time. The results suggest that multi-class models can be more accurate than single-class models, and leverage inter-class relationships.
We then investigate DL models for registration of pMR with iUS images. Image registration is inherently difficult, and especially with different modalities such as MR and US. In addition, there is a high variability across cases, as different structures are visible in the limited US field of view, depending on tumor location. It is also difficult to estimate ground truth displacements for training DL models. While we were not able to train a registration model with the available data, we discuss our findings and possible areas of improvement.
DL models can successfully segment iUS images. We currently lack data to train registration models but expect that larger datasets will enable the training of such models.