PhD defense of Basile JUMENTIER on 06/09/22

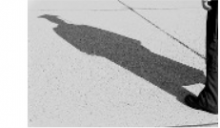
PhD defense of Basile JUMENTIER from TIMC BCM on June, the 9th, at 2pm:
« Analysis of the mediation of effects of environmental exposures on health via DNA methylation:
Application to prenatal exposure to tobacco and air pollution and child health»
-
Place: Amphithéâtre Supérieur Nord du bâtiment Jean Roget, Faculté de Médecine et Pharmacie de Grenoble, 23 avenue des Maquis du Grésivaudan, 38700 La Tronche
Jury:
- Olivier FRANÇOIS, Enseignant Chercheur, Grenoble INP-Université Grenoble Alpes, Director
- Johanna LEPEULE, Chercheuse, INSERM U1209-Environmental epidemiology, Grenoble, Co-director
- Vivian VIALLON, Maître de Conférence, Université Claude Bernard Lyon, Reporter
- Mathieu EMILY, Professeur, Institut Agro - Agrocampus Ouest, Reporter
- Vincent BONNETERRE, Professeur des Universités - Praticien Hospitalier, Université Grenoble Alpes, Examiner
- Magali RICHARD, Maîtresse de Conférence, Université Grenoble Alpes, Examiner
Keywords:
Prenatale exposure, Child health, Child health
Abstract:
High-dimensional mediation analysis is an extension of one-dimensional mediation analysis that includes multiple mediators and is increasingly used in environmental epidemiology to assess indirect epigenetic effects of environmental exposures on health outcomes. However, analyzes involving high-dimensional data raise several statistical issues. Although many methods have recently been developed to solve these problems, no consensus has been reached on an optimal combination of approaches. To better understand the problem of mediation in high dimension, the first chapter of the thesis focuses on the studies of associations such as EWAS and GWAS. Associations of phenotypes or exposures with genomic and epigenomic data face significant statistical challenges. One such challenge is accounting for variation due to unobserved confounders, such as individual ancestry or cell type composition in tissues. This problem can be solved with penalized latent factor regression models, where penalties are introduced to deal with a removed dimension in the data. If a relatively small proportion of genomic or epigenomic markers is correlated with the variable of interest, sparse penalties may help capture relevant associations, but improvement over non-parsimonious approaches has yet to be seen fully assessed. Here, we present least squares algorithms that jointly estimate effect sizes and confounders in sparse latent factor regression models. In simulated data, sparse latent factor regression models generally obtained better statistical performance than other sparse methods, such as LASSO (Least Absolute Shrinkage and Selection Operator) and BSLMM (Bayesian Sparse Linear Mixed Model). In generative model simulations, statistical performance was slightly lower (but comparable) to non-parsimonious methods, but in simulations based on empirical data, parsimonious latent factor regression models were more robust than non-parsimonious approaches. We applied sparse latent factor regression models to a genome-wide association study of a flowering trait of the plant Arabidopsis thaliana and an epigenome-wide association study of the smoking status in pregnant women. For both applications, sparse latent factor regression models facilitated the estimation of non-zero effect sizes while overcoming multiple testing issues. The results were not only consistent with previous findings, but also identified novel genes with functional annotations relevant to each application. In the second chapter, we developed HDMAX2, a novel multi-step mediation approach that combines latent factor regression models for epigenome-wide association studies with mediation tests (maximum squared). HDMAX2 has been carefully evaluated from simulations and compared to high-dimensional mediation methods. Then, HDMAX2 was used to assess the indirect effects of maternal smoking exposure on term birth weight and gestational age at delivery in a study of 470 women from the EDEN mother-child cohort.
During HDMAX2 simulations it is shown to be more powerful compared to existing high-dimensional mediation methods. It made it possible to detect regions not identified in previous analyzes of the mediation of exposure to smoking on birth weight. The results provided evidence for a polygenic architecture of the causal pathway with an overall indirect effect of 44 g lower body weight (31% of the total effect size). HDMAX2 also identified regions with simultaneous effects on both gestational age and birth weight. Among the main findings of the gestational age and birth weight analyses, regions located on the COASY and BLCAP genes also mediated the relationship between gestational age and birth weight, suggesting reverse causation in the relationship between gestational age and methylome.